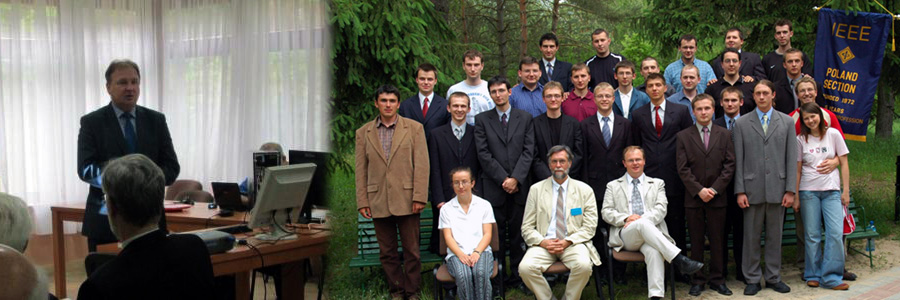
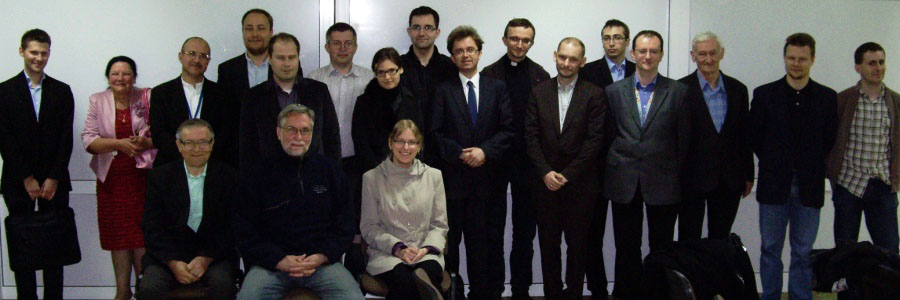
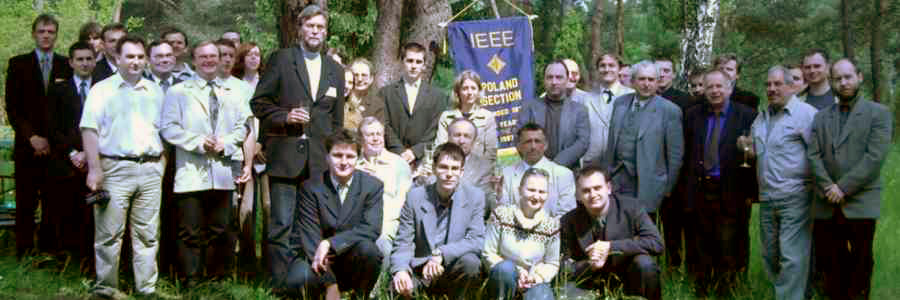
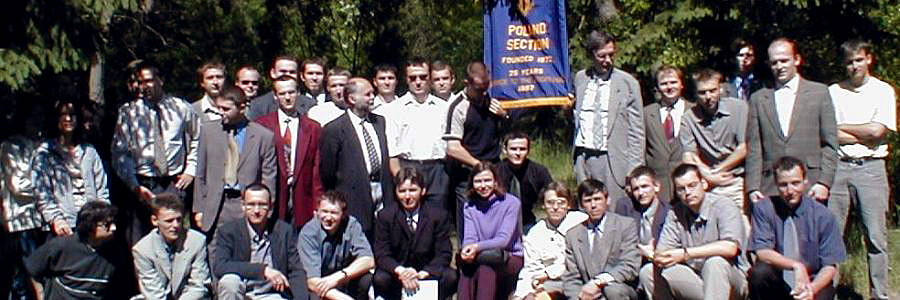
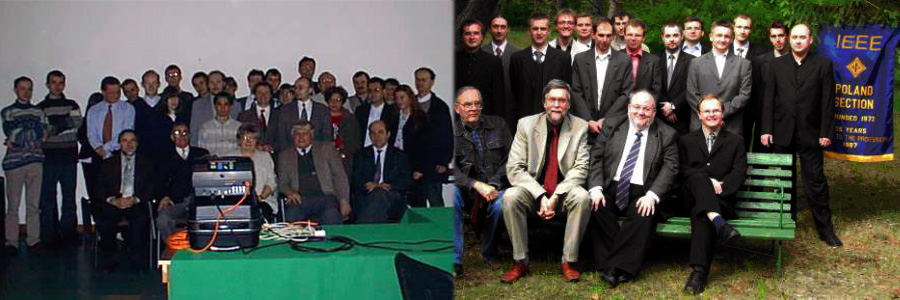
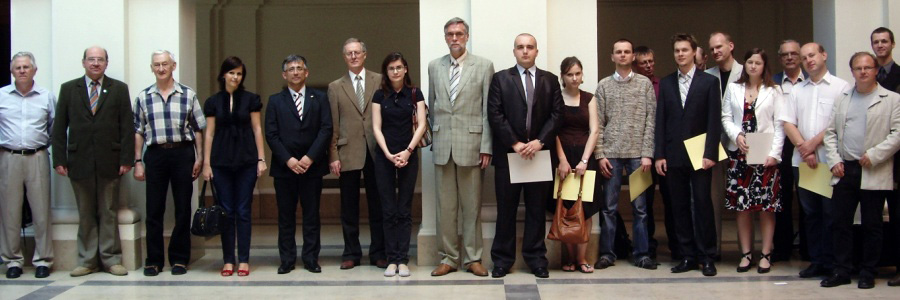
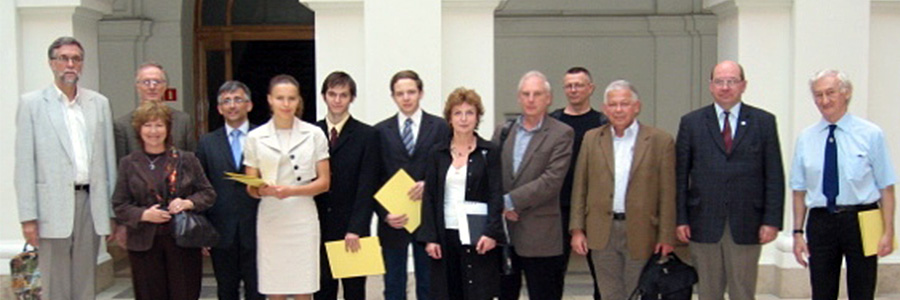
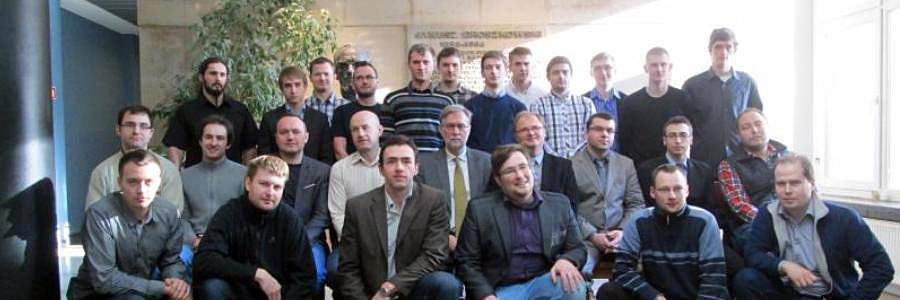
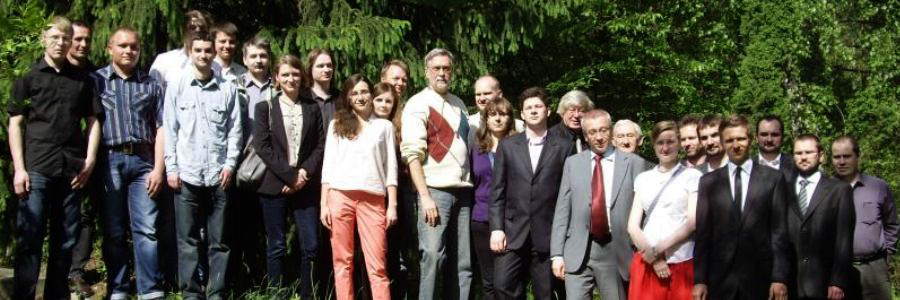
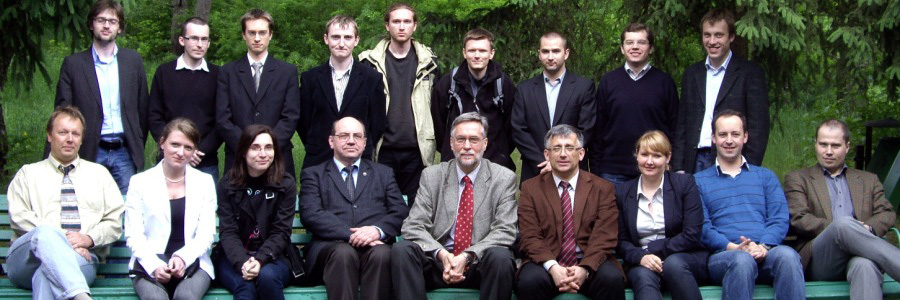
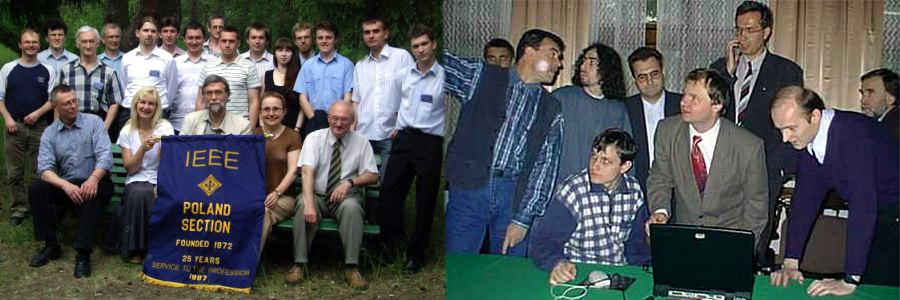
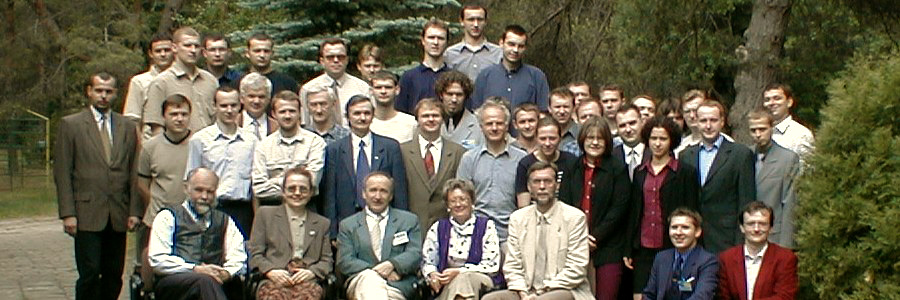
Exploiting random perturbations to defend against adversarial attacks
Adversarial examples are deliberately crafted data points which aim to induce errors in machine learning models. This phenomenon has gained much attention recently especially in the field of image classification and many methods have been proposed to generate such malicious examples. In this paper we focus on defending a trained model against such attacks by introducing randomness to it's inputs.
Author: Paweł ZawistowskiConference: Title