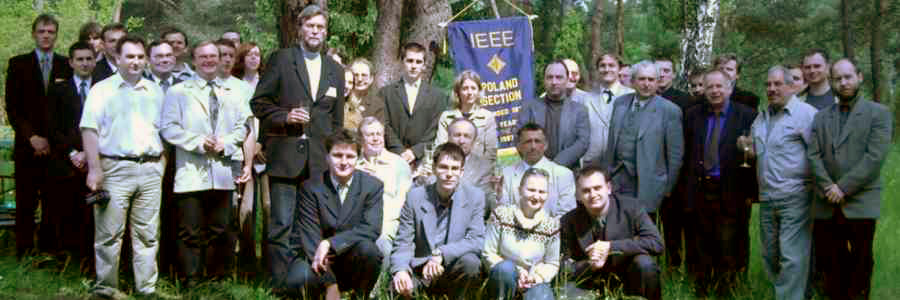
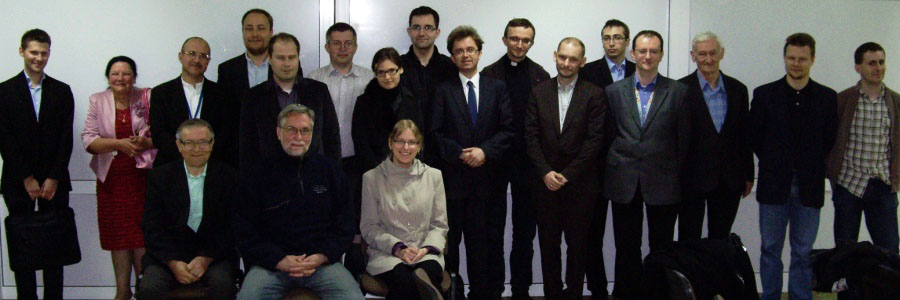
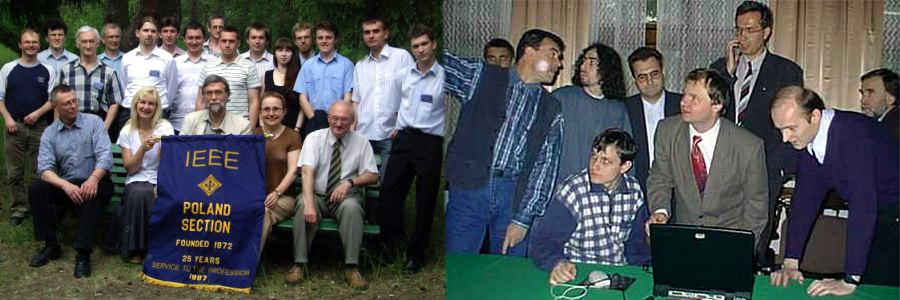
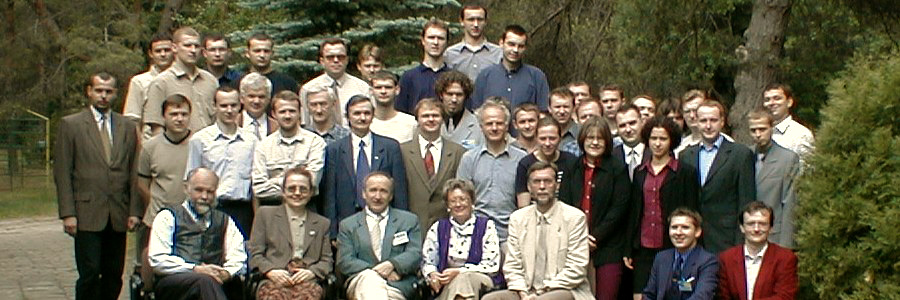
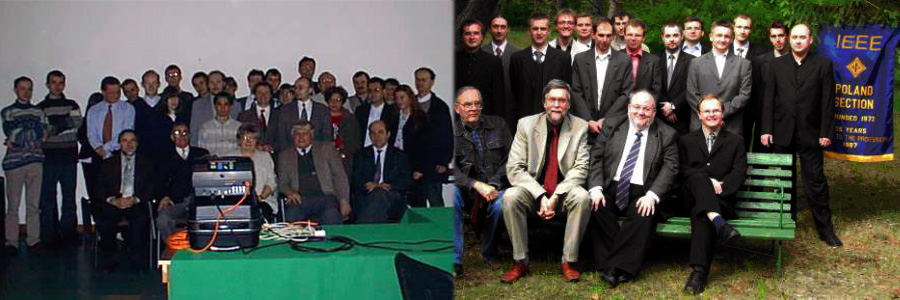
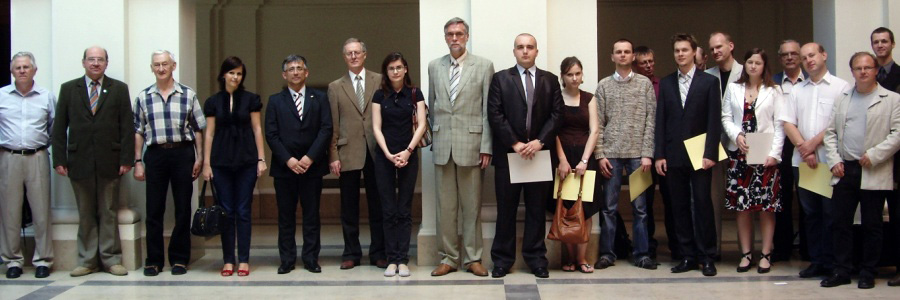
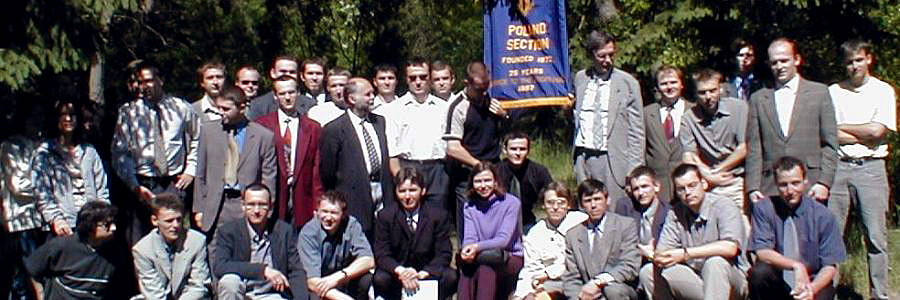
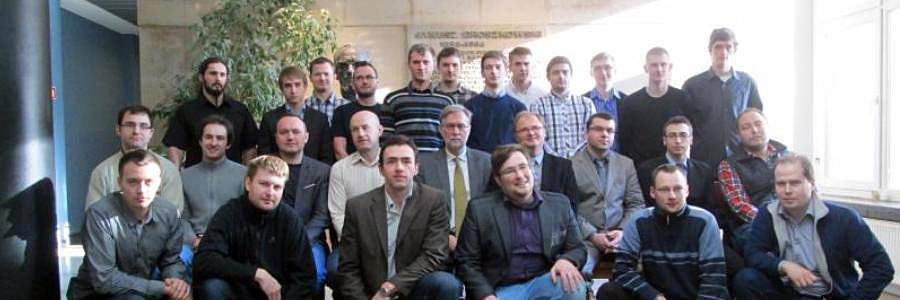
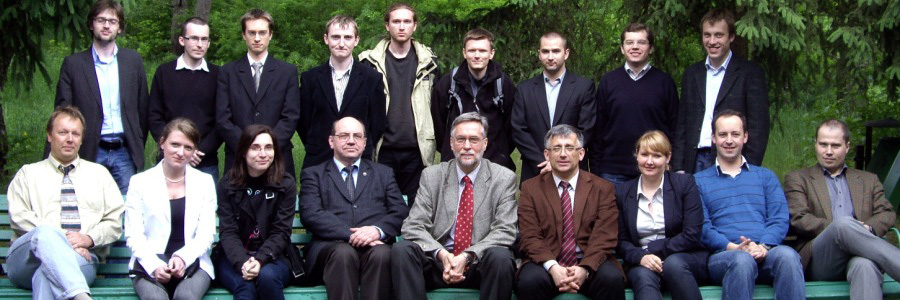
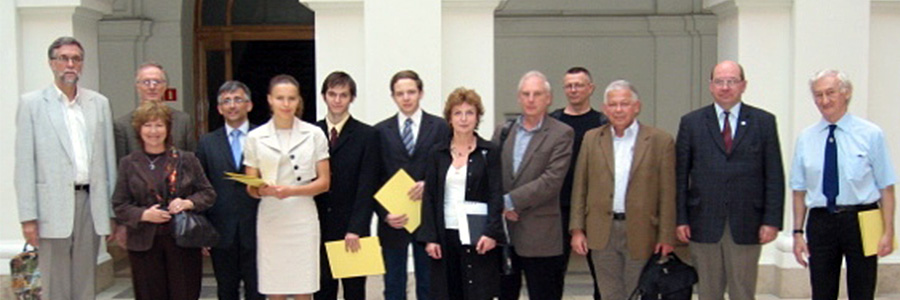
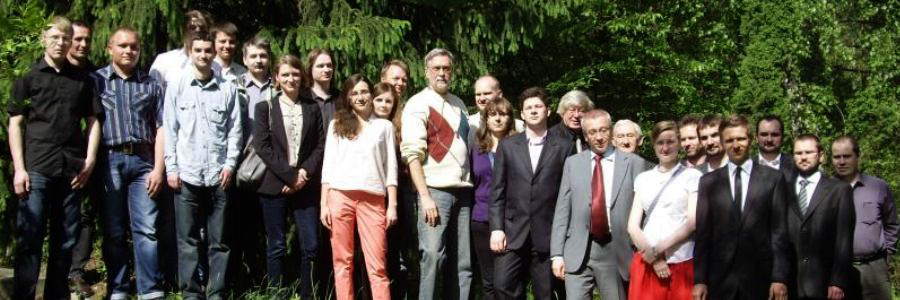
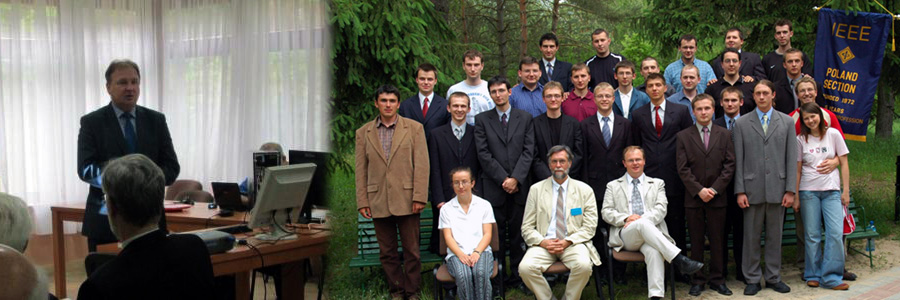
Common CNVs detection by artificial intelligence methods
Many organisms, in particular people, contain sections of the genome which could be present in various number of copies between individuals. This event is called copy number variations (CNVs) and in many cases is associated with genetic diseases. However, the accuracy of CNV detection in the human genome is still low.
We propose the new algorithm for common CNVs detection based on artificial intelligence algorithms. We generalized a common CNVs detection task to classification problem. In this paper we showed some classification models and compare them in order to detect common CNVs.
The algorithm contains three stages: counting depth of coverage in targets (whole exome sequencing), quality control of targets and training the models. Then, trained models are used to detetct CNVs in a new sample.
The proposed approach was tested, the obtained CNVs calls showed the corecctness of our proposals. The results present, that our approach is designed to detect only common CNVs, the sensitivity and specificity of the approach are higher than for another algorithms. However, rare CNVs are not discovered, but we plan to extend presented approach in order to detect also rare CNVs (based on anomalies detection algorithms).
The presented approach could improve the accuracy of detection common CNVs in the human genome. The described method could be useful in labolatories, where large volume of annotated common CNVs dataset exists. What is more, to our knowledge, this is the first paper which shows the usage of artificial intelligence methods in common CNVs detection problem.
Author: Wiktor Kuśmirek